How to Use AI Properly
Understanding The Fundamentals of AI
As you begin to integrate Artificial Intelligence (AI) into your daily life and work, it’s imperative to understand how to use it effectively and responsibly. With great power comes great responsibility, and AI is no exception. Improper use of AI can lead to biased results, data breaches, and even job displacement. On the other hand, when used correctly, AI can streamline processes, enhance decision-making, and unlock new opportunities. In this tutorial, you’ll learn how to harness the power of AI while avoiding common pitfalls and ensuring transparency, accountability, and ethics in your AI-driven endeavours.
Key Takeaways:
Here are the top 5 key takeaways on how to use AI properly:
- Define Clear Objectives: Before implementing AI, identify specific business problems or opportunities you want to address. Clearly define what you want to achieve with AI, and ensure your objectives are measurable and achievable.
- Data Quality Matters: AI models are only as good as the data they’re trained on. Ensure your data is accurate, complete, and relevant to the problem you’re trying to solve. Poor data quality can lead to biased or inaccurate results.
- Choose the Right AI Tool: With numerous AI tools available, select one that aligns with your objectives and data type. Consider factors like scalability, integrations, and user experience to ensure the tool meets your needs.
- Monitor and Evaluate AI Performance: Continuously monitor AI model performance and evaluate its impact on your business. This helps identify biases, errors, or areas for improvement, ensuring AI is used responsibly and effectively.
- Human Oversight is Crucial: AI should augment human decision-making, not replace it. Ensure humans are involved in the decision-making process, especially when it comes to high-stakes or sensitive applications, to prevent unintended consequences.
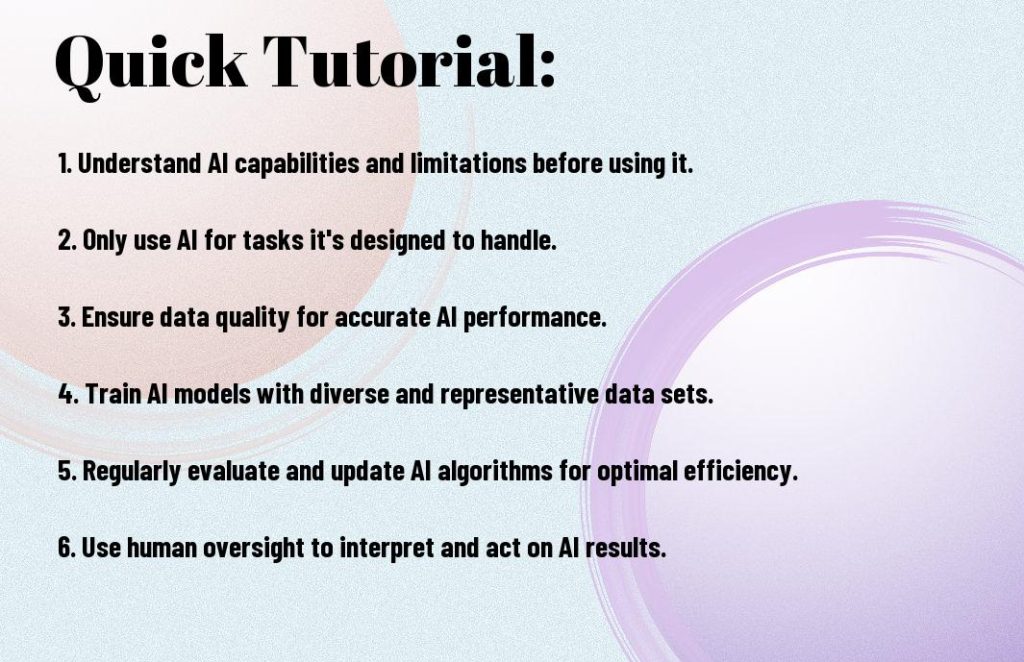
Understanding AI Fundamentals
To use AI properly, you need to start with the basics. Understanding AI fundamentals is crucial to harnessing its power and avoiding potential pitfalls.
What is Artificial Intelligence?
To grasp the concept of AI, you need to understand what it is and how it works. Artificial intelligence refers to the development of computer systems that can perform tasks that typically require human intelligence, such as learning, problem-solving, and decision-making. These systems use algorithms and data to make predictions, classify objects, and generate insights.
The key characteristic of AI is its ability to process and analyze vast amounts of data quickly and accurately, often surpassing human capabilities. This enables AI systems to automate tasks, optimize processes, and provide valuable insights that can inform business decisions or improve daily life.
Types of AI: Narrow, General, and Superintelligence
If you’re new to AI, you might be wondering about the different types of AI. There are three main categories: Narrow or Weak AI, General or Strong AI, and Superintelligence.
A narrow AI system is designed to perform a specific task, such as facial recognition, language translation, or playing chess. General AI, on the other hand, refers to a system that can perform any intellectual task that a human can. Superintelligence is an AI system that significantly surpasses human intelligence, potentially leading to exponential growth in technological advancements.
- Narrow AI: designed to perform a specific task
- General AI: can perform any intellectual task that a human can
- Superintelligence: significantly surpasses human intelligence
Assume that you’re building an AI system to analyze customer feedback. You would use narrow AI to develop a system that can classify feedback as positive, negative, or neutral.
Type of AI | Description |
Narrow AI | Designed to perform a specific task |
General AI | Can perform any intellectual task that a human can |
Superintelligence | Significantly surpasses human intelligence |
Hybrid AI | Combines different AI approaches to achieve a goal |
A key aspect of understanding AI fundamentals is recognizing the differences between these types of AI. By doing so, you can determine the best approach for your specific use case and avoid potential risks associated with more advanced forms of AI.
Important note: As you explore AI, keep in mind that the development and use of superintelligence raise significant ethical concerns. It’s crucial to consider the potential consequences of creating an AI system that surpasses human intelligence.
Setting AI Goals and Objectives
Assuming you have a good understanding of the AI landscape and its potential applications, the next step is to set clear goals and objectives for your AI project. This is a crucial stage, as it will determine the direction and focus of your project.
Defining AI Project Scope and Requirements
Delineating the scope of your AI project involves identifying what you want to achieve and what problems you want to solve. Be specific about what you want to accomplish, and prioritize your objectives. Ask yourself questions like: What are the key business challenges you want to address? What are the opportunities you want to capitalize on? What are the must-haves, nice-to-haves, and nice-to-have-but-not-necessary requirements for your project? By answering these questions, you’ll be able to define a clear scope for your project and ensure everyone involved is on the same page.
It’s also necessary to identify the key stakeholders involved in your project and their expectations. Who will be using the AI system, and what are their needs and pain points? What are the technical requirements for your project, such as data storage, processing power, and integration with existing systems? By understanding these requirements, you’ll be able to develop an AI solution that meets the needs of your organization and stakeholders.
Identifying Key Performance Indicators (KPIs)
With clear goals and objectives in place, you need to establish metrics to measure the success of your AI project. KPIs will help you track progress, identify areas for improvement, and make data-driven decisions. Examples of KPIs for AI projects include accuracy rates, processing times, and return on investment (ROI).
To ensure the effectiveness of your KPIs, make sure they are specific, measurable, achievable, relevant, and time-bound (SMART). For instance, instead of setting a vague goal like “improve customer satisfaction,” set a specific KPI like “increase customer satisfaction ratings by 15% within the next six months.” This will give you a clear target to work towards and help you stay focused on what matters most.
To further emphasize the importance of KPIs, not having clear metrics can lead to project failure, as it’s difficult to determine whether the AI system is meeting its intended objectives. On the other hand, well-defined KPIs can help you identify areas for improvement and optimize your AI system for better performance. By setting the right KPIs, you’ll be able to measure the success of your AI project and make data-driven decisions to drive business value.
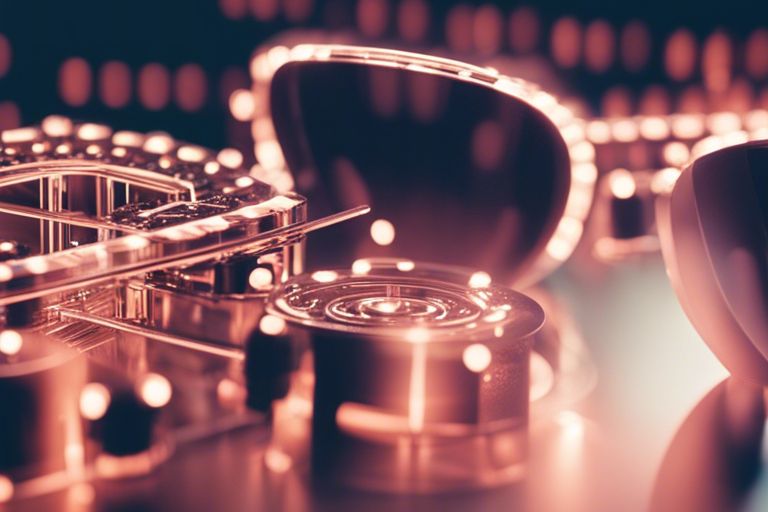
Data Preparation and Management
All successful AI projects begin with high-quality data. As you commence on your AI journey, it’s necessary to understand the importance of data preparation and management. According to an article on LinkedIn, Unleashing the Potential of AI in Education, “AI systems are only as good as the data they’re trained on.” This statement highlights the critical role data plays in AI development.
Collecting and Preprocessing Data
Data collection is the first step in preparing your data for AI. You’ll need to gather relevant data from various sources, such as databases, files, or external APIs. **Be cautious when collecting data from external sources**, as it may contain biases or inaccuracies that can affect your AI model’s performance. Once you’ve collected your data, preprocessing is necessary to ensure it’s in a format suitable for AI algorithms. This involves cleaning, transforming, and normalizing your data to remove any inconsistencies or errors.
During preprocessing, you may need to handle missing values, remove duplicates, or convert data types. **It’s crucial to document your preprocessing steps** to maintain transparency and reproducibility in your AI project. By doing so, you’ll be able to track any changes made to your data and ensure that your AI model is trained on high-quality data.
Data Quality and Integrity
Assuming your data is accurate and reliable is a common mistake many AI practitioners make. However, **data quality issues can lead to biased or inaccurate AI models**, which can have severe consequences in real-world applications. It’s necessary to validate your data against a set of quality metrics, such as accuracy, completeness, and consistency.
You should also ensure that your data is representative of the problem you’re trying to solve. **Failure to do so can result in AI models that perpetuate existing biases or discriminate against certain groups**. To avoid this, you should strive to collect diverse and inclusive data that reflects the complexity of real-world scenarios.
Plus, it’s vital to establish data governance policies to ensure data quality and integrity throughout the AI development lifecycle. This includes implementing data validation checks, performing regular data audits, and providing training to data handlers on best practices for data management.
Data Storage and Security
While data preparation and preprocessing are crucial, you must also consider the security and storage of your data. **Data breaches or unauthorized access can compromise your AI project’s integrity**, leading to reputational damage and financial losses. You should store your data in secure databases or repositories, using encryption and access controls to protect sensitive information.
It’s also important to implement data backup and recovery procedures to ensure business continuity in the event of data loss or corruption. **Regular security audits and penetration testing** can help identify vulnerabilities in your data storage systems, allowing you to take proactive measures to prevent data breaches.
A well-designed data storage and security strategy is critical to the success of your AI project. By prioritizing data security and integrity, you can build trust with your stakeholders and ensure that your AI model is trained on high-quality, reliable data.
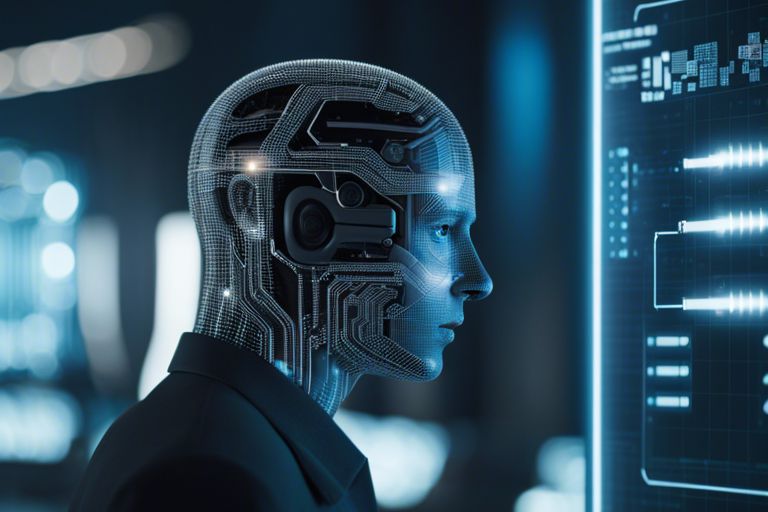
Choosing the Right AI Tools and Technologies
Despite the numerous benefits of AI, selecting the right tools and technologies can be overwhelming, especially for those new to the field. With so many options available, it’s imperative to understand what you need and how to choose the best fit for your project.
Overview of Popular AI Frameworks and Libraries
Choosing an AI framework or library can significantly impact the success of your project. Popular frameworks like TensorFlow, PyTorch, and Keras offer pre-built functionality and extensive communities, making it easier to develop and deploy AI models. Libraries like OpenCV and scikit-learn provide efficient implementations of various AI algorithms, allowing you to focus on building your application. Understanding the strengths and weaknesses of each framework and library is crucial to selecting the right one for your project.
For instance, TensorFlow is ideal for large-scale deep learning projects, while PyTorch is better suited for rapid prototyping and dynamic computation graphs. Keras, on the other hand, provides an easy-to-use interface for building neural networks. By understanding the unique characteristics of each framework and library, you can make an informed decision that aligns with your project’s requirements.
Selecting the Best AI Model for Your Project
Any AI project begins with selecting the right model. With numerous models available, it’s imperative to evaluate your project’s requirements and choose a model that aligns with your goals. Consider factors like model complexity, training time, and accuracy to ensure you select a model that meets your needs.
You should also consider the type of problem you’re trying to solve. For instance, if you’re working on a computer vision project, a convolutional neural network (CNN) might be the best choice. If you’re working on a natural language processing (NLP) project, a recurrent neural network (RNN) or transformer model might be more suitable. Note, selecting the right model is critical to achieving success in your AI project.
Frameworks like TensorFlow and PyTorch provide pre-built estimators and models that can be used as a starting point for your project. These models can be fine-tuned to suit your specific needs, saving you time and resources. By leveraging these pre-built models, you can accelerate your project’s development and achieve better results.
Evaluating AI Vendor Solutions
Choosing an AI vendor solution can be a daunting task, especially with so many options available. It’s imperative to evaluate each solution based on your project’s requirements and goals. Consider factors like scalability, customization, and integration with existing systems to ensure you select a solution that meets your needs.
You should also evaluate the vendor’s expertise and support. Look for vendors with a proven track record of delivering successful AI projects and providing ongoing support and maintenance. This will ensure that you receive the necessary guidance and resources to achieve success in your project.
Overview of the vendor’s technology stack and architecture is also important. Make sure the vendor’s solution aligns with your existing infrastructure and can integrate seamlessly with your systems. Be wary of vendors with proprietary solutions that may lock you into their ecosystem. By carefully evaluating each vendor solution, you can make an informed decision that aligns with your project’s requirements and goals.
Building and Training AI Models
Keep in mind that building and training AI models is a crucial step in using AI properly. This process requires careful planning, attention to detail, and a deep understanding of your goals and data.
Designing and Developing AI Models
To create an effective AI model, you need to define its purpose and objectives clearly. Identify the problem you want to solve and determine what kind of data you’ll need to collect and analyze. This will help you choose the right algorithm and architecture for your model. Be mindful of, a well-designed model is vital for achieving accurate results and avoiding bias.
When developing your AI model, ensure that you’re using high-quality data that’s relevant to your problem domain. Data quality is critical, as poor data can lead to inaccurate or biased results. You should also consider factors like scalability, interpretability, and explainability when designing your model.
Training and Testing AI Models
Now that you have a well-designed model, it’s time to train and test it. This involves feeding your model a large dataset and adjusting its parameters to minimize errors and improve performance. Be careful not to overfit or underfit your model, as this can lead to poor generalization and inaccurate results.
During the testing phase, you’ll evaluate your model’s performance using metrics like accuracy, precision, and recall. This will help you identify areas for improvement and refine your model further. It’s vital to use a separate testing dataset to ensure that your model generalizes well to new, unseen data.
Designing a robust testing framework is critical to ensure that your model performs well in real-world scenarios. This includes testing for edge cases, handling outliers, and evaluating your model’s performance on diverse datasets.
Model Evaluation and Optimization
Any AI model can be improved with ongoing evaluation and optimization. This involves continuously monitoring your model’s performance, identifying areas for improvement, and refining its parameters to achieve better results. Regular evaluation is crucial to ensure that your model remains accurate and relevant over time.
You can use techniques like hyperparameter tuning, regularization, and ensemble methods to optimize your model’s performance. Be cautious not to over-optimize, as this can lead to overfitting and poor generalization. It’s vital to strike a balance between model performance and interpretability.
Training an AI model is an iterative process that requires ongoing evaluation and refinement. By continuously monitoring your model’s performance and making adjustments as needed, you can ensure that it remains accurate, reliable, and effective over time.
Implementing and Integrating AI Solutions
After selecting the right AI solution for your organization, it’s time to implement and integrate it into your existing systems and infrastructure. This chapter will guide you through the process of deploying AI models in production environments, integrating AI with existing systems and infrastructure, and ensuring AI solution scalability and flexibility.
Deploying AI Models in Production Environments
Some of the most critical considerations when deploying AI models in production environments are data quality, model accuracy, and security. You must ensure that your AI model is trained on high-quality data that is relevant to your specific use case. Additionally, you should regularly monitor and update your model to maintain its accuracy and prevent bias. Furthermore, you must implement robust security measures to protect your AI model and data from cyber threats. When deploying AI models in production environments, it’s vital to consider the infrastructure requirements, such as computing power, storage, and networking. You should also ensure that your AI model is compatible with your existing infrastructure and can scale to meet growing demands.
Integrating AI with Existing Systems and Infrastructure
Clearly, integrating AI with existing systems and infrastructure is crucial for seamless operations and maximum ROI. You should assess your current systems and identify areas where AI can augment or replace existing processes. You must also ensure that your AI solution is compatible with your existing systems and can integrate with them seamlessly. Models require data to function effectively, and integrating AI with existing systems and infrastructure enables the flow of data between systems. This integration allows you to leverage your existing data and systems, reducing the need for duplicate data entry and minimizing errors. Moreover, integrating AI with existing systems and infrastructure enables you to automate workflows, reduce manual intervention, and increase efficiency. This integration can also help you identify areas where AI can replace or augment human decision-making, freeing up your team to focus on higher-value tasks.
Ensuring AI Solution Scalability and Flexibility
Little do many organizations realize that scalability and flexibility are critical factors in the long-term success of AI solutions. You should design your AI solution to scale with your growing business needs, ensuring that it can handle increased data volumes, user traffic, and computational requirements. To ensure scalability and flexibility, you should consider cloud-based infrastructure, containerization, and microservices architecture. You should also implement automated testing, monitoring, and deployment processes to ensure that your AI solution can adapt to changing requirements. With the right approach to scalability and flexibility, you can future-proof your AI solution and ensure that it remains a valuable asset to your organization. This will enable you to respond quickly to changing market conditions, customer needs, and technological advancements.
Monitoring and Evaluating AI Performance
Not only is it vital to implement AI solutions correctly, but it’s also crucial to monitor and evaluate their performance regularly. This ensures that your AI systems are operating as intended, providing accurate results, and not perpetuating harmful biases or errors.
As you integrate AI into your workflow, remember that teaching responsible AI use is vital, not just for yourself but also for future generations. As Teaching Students to Use AI Responsibly emphasizes, it’s vital to educate users about the potential risks and benefits of AI to ensure responsible adoption.
Tracking AI Model Performance Metrics
You need to keep a close eye on your AI model’s performance metrics to identify areas for improvement. This includes tracking accuracy rates, precision, recall, F1 scores, and other relevant metrics depending on your specific use case. By regularly reviewing these metrics, you can detect any deviations from expected performance and take corrective action.
Moreover, tracking performance metrics enables you to identify opportunities to optimize your AI model for better results. This might involve refining your training data, adjusting hyperparameters, or exploring alternative algorithms. By continually monitoring and refining your AI model, you can ensure it remains effective and efficient.
Identifying and Addressing AI Bias and Errors
To prevent AI systems from perpetuating harmful biases or errors, you must proactively identify and address these issues. This involves regularly auditing your AI model’s outputs for signs of bias, such as discrimination based on race, gender, or other protected characteristics.
Additionally, you should establish procedures for reporting and addressing errors or biases when they occur. This might involve implementing human oversight and review processes to detect and correct mistakes.
Plus, it’s vital to recognize that AI bias and errors can have serious real-world consequences, such as perpetuating discrimination or causing harm to individuals. By prioritizing transparency and accountability in your AI development and deployment, you can mitigate these risks and ensure responsible AI use.
Continuously Improving AI Solution Effectiveness
Even after deploying an AI solution, your work is far from over. You need to continually assess and refine your AI system to ensure it remains effective and efficient.
This involves staying up-to-date with the latest advancements in AI research and development, as well as incorporating user feedback and insights to improve your AI solution. By embracing a culture of continuous improvement, you can ensure your AI system remains relevant and effective over time.
Tracking emerging trends and breakthroughs in AI can also help you identify opportunities to enhance your AI solution’s capabilities and stay ahead of the competition. By committing to ongoing improvement, you can unlock the full potential of AI and drive meaningful results.
Conclusion
On the whole, using AI properly requires a combination of understanding its capabilities, being aware of its limitations, and taking steps to mitigate its potential risks. You need to recognize that AI is a tool, not a replacement for human judgment and decision-making. By doing so, you can harness the power of AI to automate repetitive tasks, gain insights from data, and improve your overall productivity and efficiency.
As you launch on your AI journey, remember that it’s crucial to approach AI with a critical and nuanced mindset. You should continually evaluate the data that fuels your AI systems, ensure that they are transparent and explainable, and take steps to prevent bias and discrimination. By following these guidelines, you can unlock the full potential of AI and create a better future for yourself and others. Ultimately, the responsible use of AI depends on you, and by using it properly, you can reap its many benefits while minimizing its risks.
FAQ
Q: What are the key principles to keep in mind when using AI properly?
A: When using AI properly, it’s crucial to remember that AI systems are tools designed to augment human capabilities, not replace them. Key principles to keep in mind include being aware of AI’s limitations, understanding the data used to train the system, and recognizing potential biases. Additionally, it’s crucial to establish clear goals and objectives, monitor AI-driven decisions, and maintain transparency throughout the decision-making process. By following these principles, you can ensure responsible and effective AI adoption.
Q: How do I choose the right AI tool for my specific needs?
A: Choosing the right AI tool depends on your specific goals, industry, and requirements. Start by identifying the problem you want to solve or the opportunity you want to capitalize on. Research different AI technologies, such as machine learning, natural language processing, or computer vision, to determine which one best addresses your needs. Evaluate vendors and their products based on factors like accuracy, scalability, integration capabilities, and customer support. It’s also crucial to consider the total cost of ownership, including training, maintenance, and updates. Finally, ensure the AI tool aligns with your organization’s values and ethical standards.
Q: What are some common pitfalls to avoid when using AI?
A: When using AI, it’s crucial to avoid common pitfalls that can lead to inaccurate results, biased decisions, or even reputational damage. Some common pitfalls to avoid include overfitting or underfitting your model, failing to diversify your training data, and neglecting to monitor and update your AI system regularly. Additionally, be cautious of relying too heavily on AI-driven insights without human oversight, ignoring potential biases in the data or algorithm, and failing to establish clear accountability for AI-driven decisions. By being aware of these pitfalls, you can minimize risks and ensure responsible AI adoption.
Q: How do I ensure transparency and explainability in AI-driven decision-making?
A: Ensuring transparency and explainability in AI-driven decision-making is critical for building trust and accountability. One approach is to use techniques like model interpretability, which provides insights into how the AI system arrives at its decisions. Another approach is to implement transparent data practices, such as data provenance and audit trails, to track the origin and flow of data used in AI-driven decision-making. Additionally, consider using AI systems that provide clear explanations for their recommendations or decisions, and establish processes for human oversight and review. By prioritizing transparency and explainability, you can increase confidence in AI-driven decision-making and minimize the risk of unintended consequences.
Q: What steps can I take to mitigate the risk of AI bias and ensure fairness?
A: Mitigating the risk of AI bias and ensuring fairness requires a proactive and multi-faceted approach. Start by understanding the potential biases in your data and taking steps to address them, such as data augmentation, debiasing, or using diverse data sources. Implement robust testing and validation procedures to detect and correct biases in your AI system. Additionally, establish diverse and inclusive development teams, and engage with stakeholders from diverse backgrounds to ensure AI systems are designed with fairness in mind. Finally, consider using techniques like fairness metrics, bias detection tools, and regular auditing to monitor and address AI bias throughout the system’s lifecycle.